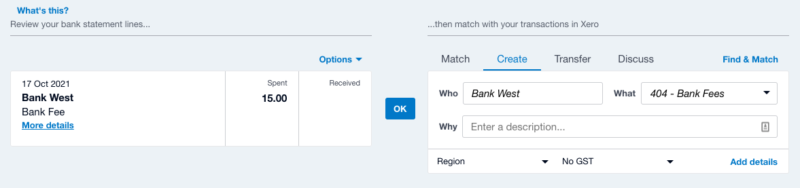
In August, we introduced our funding in machine studying to supercharge financial institution reconciliations with extra intelligence and automation. Since day dot, we’ve been centered on methods to make the routine components of operating a enterprise simpler, quicker and extra environment friendly. So that you may do not forget that we had been significantly enthusiastic about how predictive algorithms may be capable to confidently predict the contact and account code to your transactions – all by studying the patterns of previous reconciliations.
As we begin to roll out this functionality to all customers, we discovered time to sit down down with Kendra Vant, the EGM of Knowledge at Xero, to unpack how these predictive algorithms work, why we constructed them within the first place and the way Xero is utilizing all knowledge for the higher good.
Inform us a bit about this work you’re doing
We’ve been constructing machine studying algorithms to energy financial institution reconciliation predictions so we will precisely predict your transactions’ contact and account codes. This won’t solely prevent time, however cut back the handbook, hands-on work required so that you can keep in mind and log transactions appropriately. Alongside different synthetic intelligence (AI)-enabled product options like Analytics Plus and the clever form-filling in Hubdoc and Xero Bills, we’re thrilled to be taking one other step in our journey to make use of AI to assist our prospects succeed.
How will these financial institution reconciliation predictions work?
In a nutshell, our system goes to recommend the who and the what of your transaction, with the ‘who’ being the contact, and the ‘what’ being the account code. All you’ll need to do is click on ‘OK’ to reconcile the transaction totally, and it’ll even be coded to an account out of your chart of accounts. These predictions will likely be emphasised in italics that can assist you extra simply distinguish between them and common ideas, that are primarily based by yourself previous reconciled transactions.
Whereas the expertise is designed to be easy, there’s loads occurring behind-the-scenes to energy this modification. In any case, these predictions aren’t primarily based on financial institution guidelines you’ve set, or memorisations from earlier reconciliations, and even matches from current invoices and payments. As a substitute, the machine studying algorithms used to foretell a contact and account code for a transaction be taught from patterns in previous reconciliations with a purpose to present the important thing items of data required to make the reconciliation.
How are you going to predict this data with confidence?
Right here’s the place issues get actually fascinating. Consider these algorithms as having realized reconcile transactions from the collective knowledge of the entire Xero neighborhood.
For instance, if plenty of small companies purchase workplace gear from a selected place, say Officeworks or Staples, and code these transactions to ‘workplace gear’, over time our algorithms will be taught to affiliate transactions from these firms with that account code. The place this actually makes a distinction is once you see a transaction from a enterprise for the primary time – our algorithm is ready to faucet into what it has realized from years of previous reconciliations throughout Xero and predict essentially the most acceptable account code and make contact with out of your chart of accounts and make contact with listing.
Our groups are persevering with to work on enhancing the efficiency of those algorithms, and hopefully in time you’ll discover increasingly transactions getting nice predictions that prevent effort and time.
How do you educate the algorithms whereas conserving my knowledge protected?
This can be a actually necessary query, and one we take very significantly right here at Xero. It is perhaps useful to consider it like this: whereas we’re pulling from broader logic, you’ll solely ever see account or contact data from codes particular to your organisation. Conserving your knowledge protected and personal is our highest precedence and one in all our accountable knowledge use commitments.
When making a prediction, we solely use the chart of accounts, contact listing, and transaction data from your personal organisation. Which means though the machine studying algorithm has used thousands and thousands of reconciled transactions to be taught, it may well solely really predict an account code out of your chart of accounts, and a contact from both your contact listing, or utilizing phrases within the transaction description that it’s making a prediction for.
Why are these predictions necessary to folks?
We consider that any manner we will save our customers time on their financial institution reconciliations is a worthy pursuit. When companies have their accounts totally reconciled, the profit is far-reaching – they take pleasure in up-to-date experiences, extra time for increased worth enterprise actions (or their private hobbies) and tax time turns into a lot simpler as effectively. The act of reconciling is time-consuming, so it’s our hope that these predictions will assist to lighten the psychological load of attempting to recollect the place a sure transaction occurred and what it was for.
Equally, we consider that this profit extends to accountants and bookkeepers, too. Once we automate the toil, they’ve time again for deeper conversations with their purchasers round their enterprise. At its coronary heart, our funding in machine studying is about empowering folks to give attention to the larger image.
Why has this automation work come about now?
My workforce has a mantra: “Save folks time and delight, with perception”. As machine studying methods mature and Xero’s buyer base grows, we’re in a novel place to harness the knowledge of crowds for the advantage of every particular person consumer. Since its inception, financial institution reconciliation has modified the sport for accounting, giving so many individuals their useful time again. Now, machine studying could make it smarter and faster. For us, this work is the evolution of one other ‘what if?’ concept coming to life – a step in our journey in direction of making financial institution reconciliation as low-touch as doable for our prospects.
What sort of different progressive stuff is your workforce engaged on?
One factor we’re actually excited to see come to fruition is how we would create seamless, low-touch experiences for patrons simply beginning off in financial institution reconciliation for the primary time. It’s an extremely thrilling time for machine studying and AI at Xero proper now, and now we have groups in New Zealand, Australia and Canada pondering huge to seek out progressive methods to ship #stunning, machine studying experiences.
Prior to now, you will have seen among the developments we’ve made to money movement forecasting and clever kind filling, and the groups behind these applied sciences are persevering with to enhance the efficiency of the algorithms that energy them. As we roll out a few of our different machine studying pushed product improvements, I’ll be leaping again within the hot-seat to speak about these as effectively.