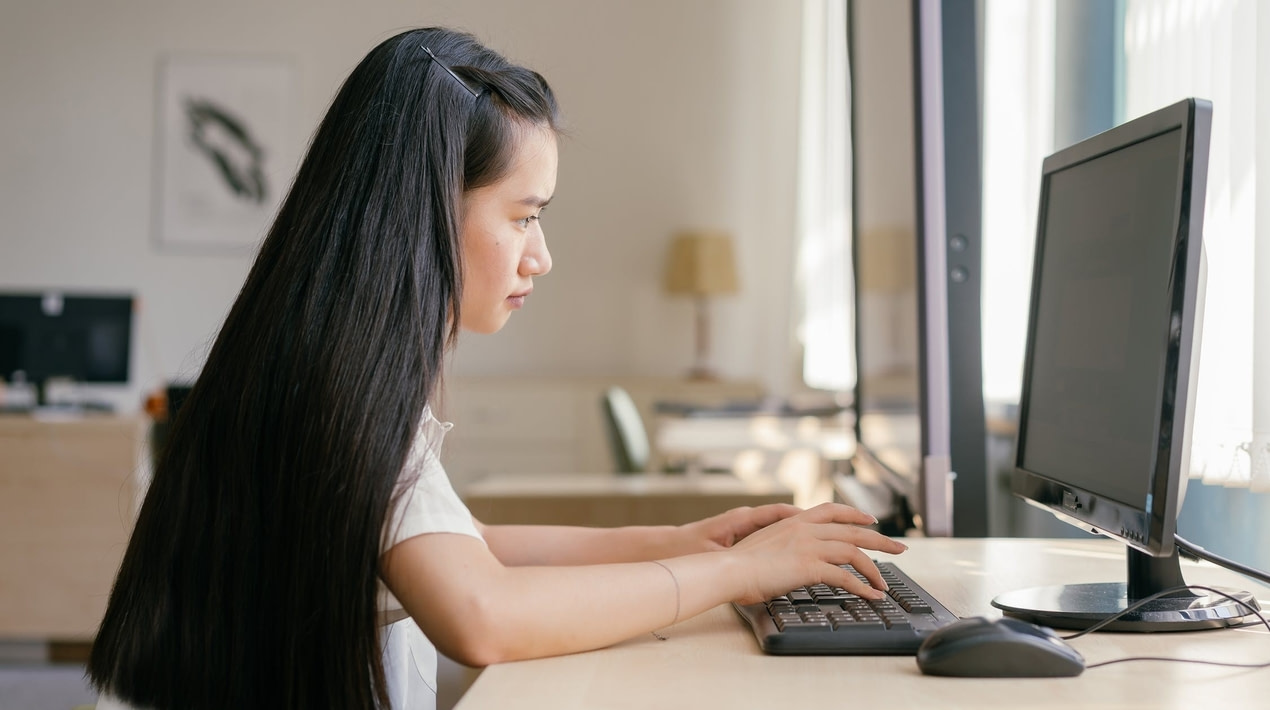
Within the wake of the pandemic, individuals internationally moved comprehensively on-line = for work, training, leisure, purchasing and monetary transactions. This has dramatically elevated the floor space for assaults and created unprecedented op[portunties for unhealthy cyber actors.
The easy reply to the challenges confronted by the monetary providers trade and different businesses is to make higher use of all accessible knowledge and superior analytics to detect and stop fraud.
In fact, this can be simpler stated and achieved. The truth is, the plethora of instruments, options and platforms accessible could make the duty extra sophisticated. The next offers some beginning factors.
Perceive the Classes of Fraud-Detection Instruments
The ‘market’ is flooded with potential options, all providing to deal with fraud. The utility of every toolset depends on the enterprise context and accessible knowledge. All should be built-in with enterprise processes and supported by coverage settings.
Here’s a brief overview that may help in mapping such instruments when it comes to their perform(s).
Detect Recognized Knowns
A watch checklist that holds details about recognized prison entities (individuals, organisations, addresses, occasions, and so forth) is an effective, common start-point.
The problem is matching the recognized entity in opposition to a brand new transaction. Easy name-matching techniques are typically shortly overwhelmed with irrelevant matches (think about looking for Mr Jones on Google – round 5,070,000,000 outcomes!).
Knowledge science can help right here by establishing a probabilistic matching system with variable threshold settings. An organisation can then match the brink settings to match its danger tolerance.
Detect Networks
The subsequent stage of detecting suspicious entities is to see the connection between a present transaction and beforehand recognized fraud. It may very well be so simple as ‘this particular person lives on the similar tackle’ to ‘the cellphone quantity used has been used to commit fraud earlier than’ and numerous variations on this theme.
Among the only community analytics techniques used for fraud detection use non-obvious knowledge. For instance, the hyperlinks could be established by connecting IP addresses, MAC codes and so forth. Among the greatest knowledge could properly reside in system logs!
Determine Patterns
Predictive fashions study accessible knowledge in opposition to recognized patterns related to fraud. At a fundamental stage, the approach can utilise easy attribute matching (eg gender, age, nationality, and so forth) however extra subtle instruments can considerably enhance the accuracy and devour a whole lot of variables.
Predictive fashions are often primarily based on knowledge analytics however additionally it is attainable to construct intelligence-based fashions when present knowledge holdings don’t help ample accuracy. The vary of processes that may fall into this class is simply restricted by knowledge availability, the talents of the info science crew and the capability to combine such techniques.
A wealthy supply of information is frequently-ignored metadata. For instance, techniques that monitor mouse
actions and keystrokes and determine potential deceit primarily based on the best way a shopper completes a web-based type can be found now.
Monitor Developments
This often-overlooked device can present early warning if there’s a variation in regular tendencies. For instance, a sudden, non-seasonal surge in refund claims from a specific area could point out the emergence of fraudulent behaviour.
Instruments that may routinely monitor pattern knowledge at international and extra granular ranges are available and generate alerts when tolerances are breached. Whereas some instruments visualise the pattern variation on a dashboard, the most effective instruments additionally generate alerts routinely and don’t depend on somebody recognizing an issue manually and even loading a dashboard.
An built-in, end-to-end, fraud detection and mitigation system could properly encompass all or a lot of these options and often requires a stage of integration with processing platforms. Thankfully, present options (eg containers) simplify the problem.
Fraud Mitigation Framework
Most authorities businesses and monetary establishments gather and keep massive volumes of information in help of their operations. Making optimum use of those knowledge collections underpins the power to determine and stop fraud.
Knowledge-driven decision-making depends on:
- with the ability to gather and see data (knowledge);
- understanding the knowledge and knowledge;
- responding with applicable counter-measures,
- monitoring/evaluating the effectiveness of those measures; and
- adjusting the system primarily based on the continual evaluation.
Seeing Data/knowledge – if it’s invisible, it’s troublesome to defeat
The flexibility to gather and retailer data and knowledge for downstream processing inside required timeframes is a elementary constructing block to any fraud-mitigation course of. Most organisations gather course of knowledge reminiscent of purposes and claims. Most would additionally retailer the outcomes of such processes (eg refused software/declare, authorised software/declare).
An organisation that information incidents of recognized malpractice in such purposes and claims creates a strong anti-fraud dataset.
Most knowledge techniques have a tendency to gather huge volumes of meta-data like system logs. A lot of this useful resource is usually saved and never successfully makes use of to detect fraud. Instruments that gather transaction metadata (eg mouse actions, keystrokes) and feed synthetic intelligence that may precisely predict doubtlessly fraudulent intent.
Capturing contextual data for evaluation offers further attributes that may improve recognized fraud however may additionally present priceless intelligence round present however undetected fraud.
Understanding – ‘why’, ‘how’, ‘when’, ‘the place’ and ‘what’ occurred
Evaluation of information and intelligence can reveal how the varied fraudulent strategies work. Typically, this depends on a crew of subject material consultants working with knowledge science groups to develop deep insights.
Responding – see when suspicious issues are taking place and cease them
As soon as the fraudulent strategies are understood, an information science crew can construct predictive analytics fashions to detect the antagonistic patterns within the knowledge to flag comparable patterns related to present (reside) transactions. Such fashions can handle a whole lot of variables in near real-time and determine problematic behaviour with a recognized stage of accuracy and work in near real-time.
There are various methods of utilizing this course of to reply to potential malpractice. One easy instance is:
- Functions/claims which can be recognized as low danger by our danger techniques may be expedited. This reduces the price of processing and will increase shopper satisfaction.
- Functions/claims which can be recognized as high-risk may very well be diverted to a course of that permits extra knowledge assortment and/or larger scrutiny.
Monitoring – are countermeasures working?
As soon as a fraud detection system has been deployed the world can have modified. Finally, criminals will alter their approaches and probably develop new methodologies.
Automated monitoring of an analytics-based system is at all times fascinating as it could actually detect when anticipated accuracy or different efficiency is not being achieved. There are various the explanation why it will happen however certainly one of them is that criminals have developed new strategies and workarounds.
Monitoring the efficiency of the analytics-based system and, importantly, accumulating and analysing intelligence can shut a lot of this hole.
Adjusting – reply shortly to modified circumstances
The ultimate a part of the method closes the loop – classes learnt by way of the monitoring processes is fed again into the subsequent model of the system to refresh predictive fashions and different elements.
Why this course of?
This course of leverages knowledge and intelligence, helps steady enchancment and a capability to reply to modified circumstances. Importantly, the method maximises the capability to use essentially the most applicable measures to mitigate fraud. In lots of circumstances, a response is predicated solely on the detection of an issue. The evaluation of the issue offers insights into the strategy of operation on this case. As soon as that is understood, an evaluation of present knowledge could point out if that is an remoted case or if extra such circumstances have remained hidden.
Furthermore, it ensures that any countermeasures goal the true downside. If the issue is doubtlessly widespread, then the hassle to construct a data-driven mannequin to detect different such circumstances and a predictive mannequin to determine comparable circumstances in future transactions is warranted. Automated monitoring and suggestions loops present a stage of assurance that our answer continues to be doing what is anticipated.